
You can only manage what you measure
Data Quality Checks
In close dialog with the LEI Regulatory Oversight Committee (ROC) and the LEI issuing organizations, GLEIF has defined a set of measurable quality criteria to clarify the concept of data quality relative to the LEI population. For this, standards developed by the International Organization for Standardization have been used. By instituting a set of defined quality criteria, GLEIF has established a transparent and objective benchmark to assess the level of data quality within the Global LEI System.
GLEIF’s Data Quality Checks ensure that the provided reference data is compliant with the current State Transition and Validation Rules in the Global LEI System. These checks have been defined based on the Common Data File (CDF) formats.
Each Data Quality Check is characterized by a maturity level, quality criterion, intention and formalized description containing precondition and condition.
This set of checks constitutes the so-called Rule Setting. Each check is of type ‘If X then Y’, where X is described as a check precondition and Y is the check condition. If a record, relationship or exception does not meet the check precondition, this check is ‘not applicable’. If it passes the precondition and goes into the condition and the value does not fulfill Y, the check is considered a ‘fail’, otherwise the check is considered a ‘pass’.
GLEIF's Rule Setting
- Intention
The intention is written in plain language and summarizes the purpose of a given Data Quality Check. - Precondition
Not every Data Quality Check is applicable for all LEI Records. For example, some Data Quality Checks are only applicable for fund entities, or active entities. The precondition of each check filters out those LEI Records that are not applicable for a given check. - Condition
In every Data Quality Check certain unambiguous fail-conditions are implemented. The condition is only applied to LEI Records that passed the precondition of a given Data Quality Check.
Check Categories
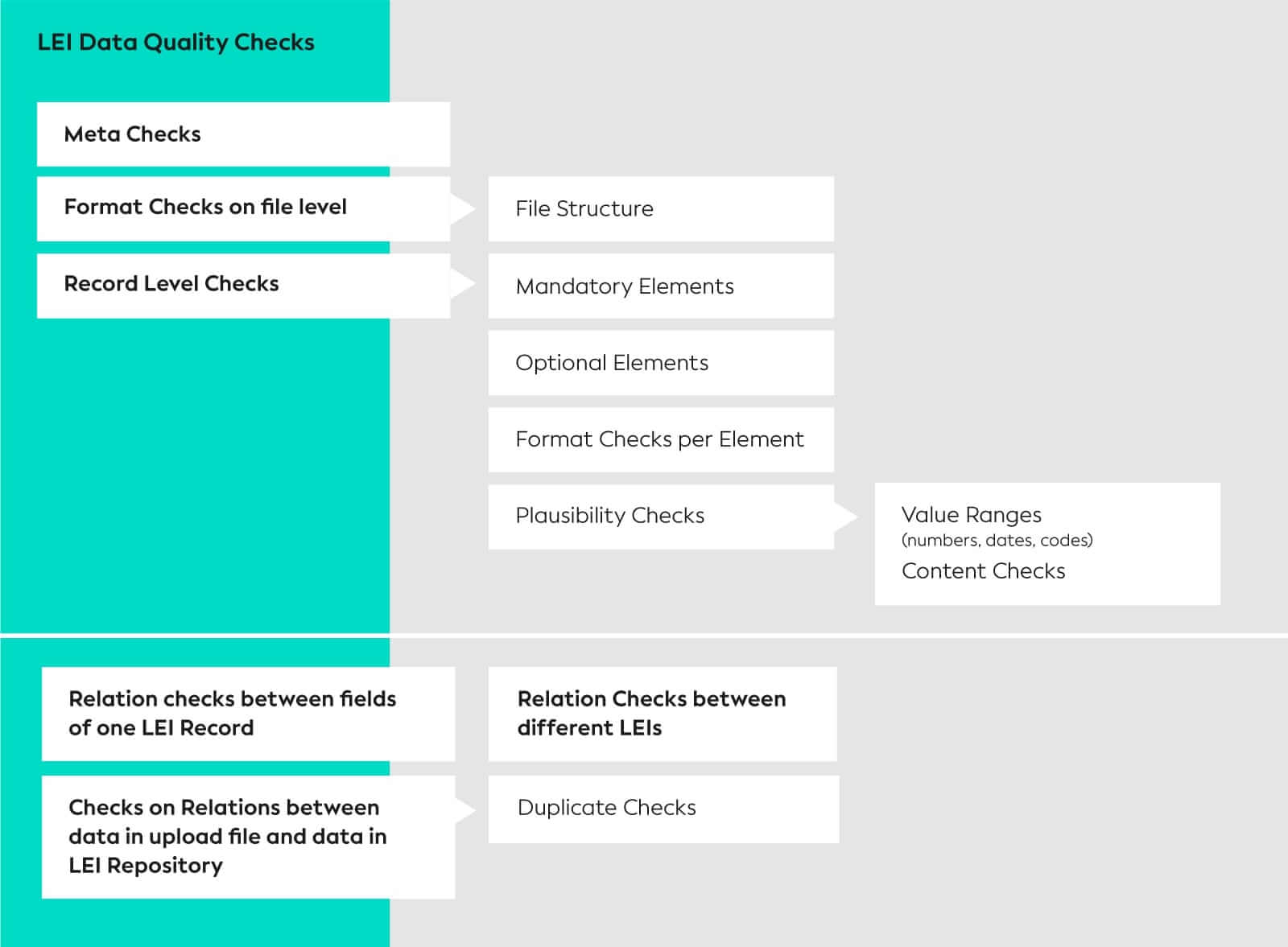
The Data Quality Checks can also be clustered in categories, based on their nature and type of measurement. For example, meta checks are focusing on the criteria of timeliness, currency and accessibility, while format checks are mostly implemented via the Common Data File formats and embedded XML Schema. Checks on the record level are applied to mandatory and optional field elements and cover format and plausibility checks (e.g. value ranges).
Maturity Level Model
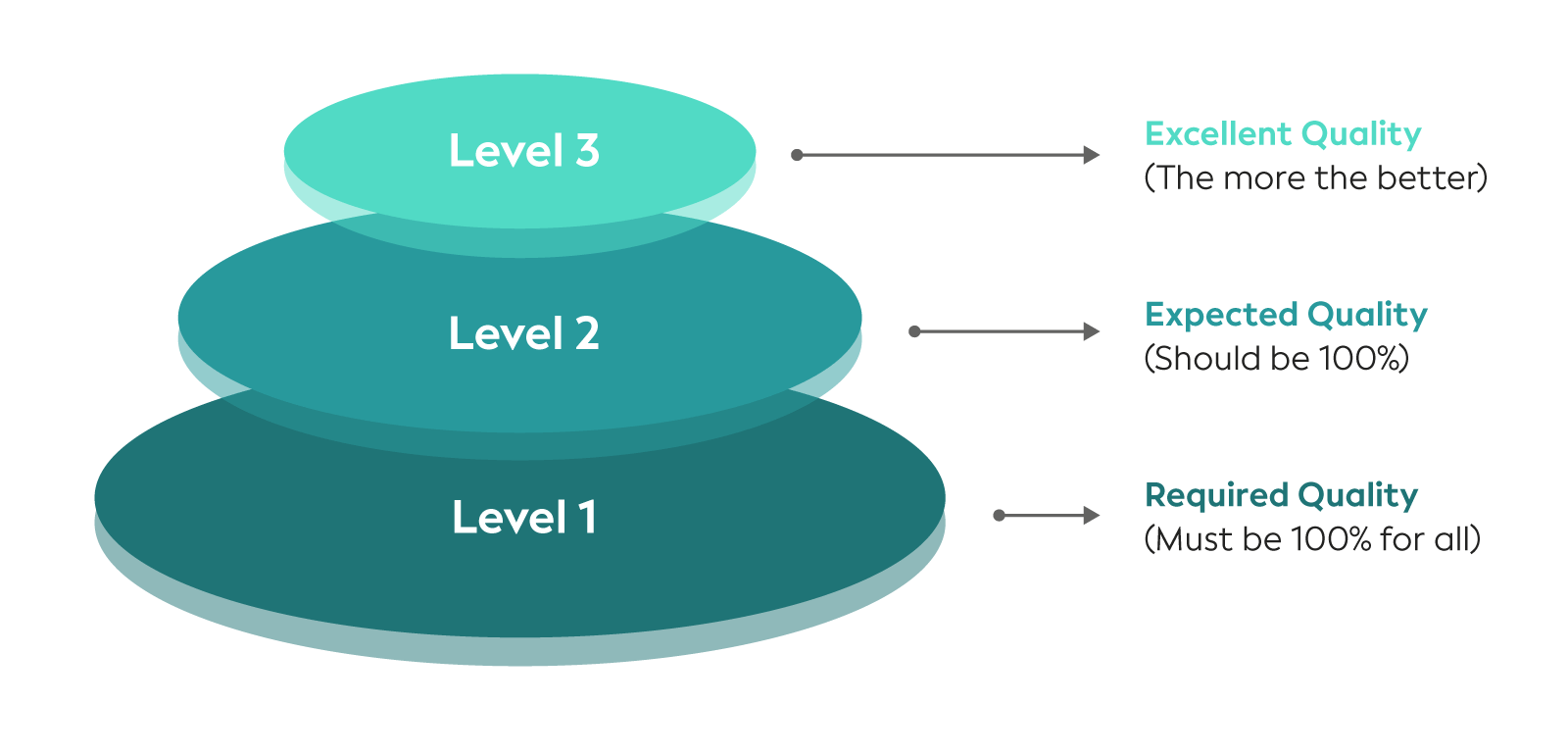
To define the Global LEI System’s overall data quality, GLEIF uses a maturity model which assesses the defined quality criteria. Based on the type of checks related to the different categories, every check is assigned to one Maturity Level. Excellent and Expected maturity levels can only be achieved if all lower levels are completely ensured.
1 - Required:
This level reflects repeatable success and consists of:
- Format checks
- All checks regarding mandatory elements
2 - Expected:
This level shows the managed success and consists of:
- Plausibility checks
- Business rule checks
- Relationship integrity checks
- All checks regarding optional elements
3 - Excellent:
The third level is that of optimized success and consists of:
- Representation checks
- Timeliness checks
- All checks regarding the LEI life-cycle and legacy records
Data Quality Criteria
By instituting a set of defined quality criteria, GLEIF has established a transparent and objective benchmark to assess the level of data quality within the Global LEI System.
Quality Criteria | Definition |
---|---|
Accuracy | The extent to which the data is free of identifiable errors / the degree of conformity of a data element or a data set to an authoritative source that is deemed to be correct or the degree the data correctly represents the truth about a real-world object |
Accessibility | The extent to which data items that are easily obtainable and legal to access with strong protections and controls built into the process |
Completeness | The degree to which all required occurrences of data are populated |
Comprehensiveness | All required data items are included, this ensures that the entire scope of the data is collected with intentional limitations documented |
Consistency | The degree to which a unique piece of data holds the same value across multiple data sets |
Currency | The extent to which data is up-to-date; a data value is up-to-date if it is current for a specific point in time, and it is outdated if it was current at a preceding time but incorrect at a later time |
Integrity | The degree of conformity to defined data relationship rules (e.g., primary/foreign key referential integrity) |
Provenance | The history or pedigree of a property value |
Representation | The characteristic of Data Quality that addresses the format, pattern, legibility, and usefulness of data for its intended use |
Timeliness | The degree to which data is available when it is required / concept of data quality that involves whether the data is up-to-date and available within a useful time frame; timeliness is determined by manner and context in which the data is being used |
Uniqueness | The extent to which all distinct values of a data element appear only once |
Validity | The measure of how a data value conforms to its domain value set (i.e., a set of allowable values or range of values) |
Relevant Files for Download
- Download as PDF: Data Quality Rule Setting v3.4
- Download as XLS: Data Quality Rule Setting v3.4
- Download PDF: State Transition and Validation Rules v2.8.4
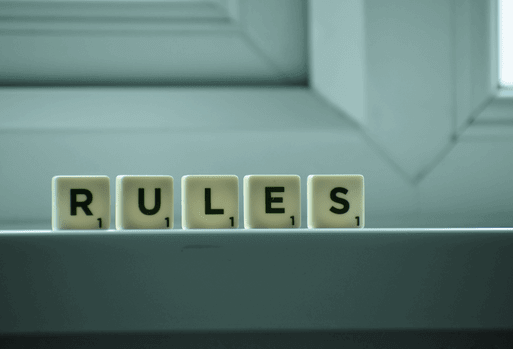
Discover GLEIF’s Rule Setting
GLEIF aims for maximum transparency and therefore publishes each Data Quality Check Specification
Read more
Data Quality Reports
GLEIF makes available Global and LEI Issuer Data Quality Reports on a monthly basis
Read more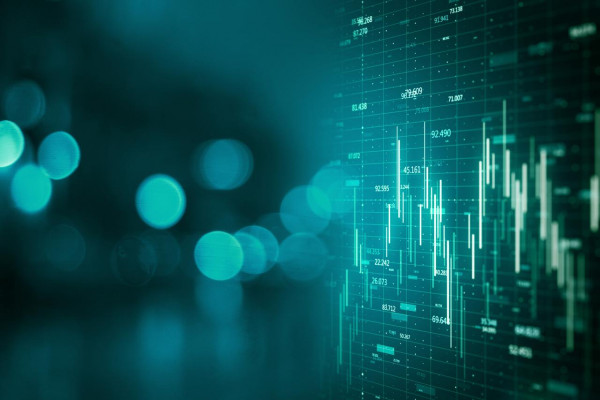
Data Quality Monitoring
GLEIF uses state-of-the-art tools and technologies to ensure continuous monitoring
Read more